Explained: AI + Health Literacy |
Will Large Language Models Ever Really Be Trustworthy Enough for Health Literacy?
An Artificial Intelligence Practitioner’s Perspective on Trustworthiness and Responsibility, Part 4
By Temese Szalai | December 7, 2023
Read more in the series:
Part 1 | Can — and Should — We Trust Large Language Models for Health Literacy? | Publish Date: November 28, 2023
Part 2 | How Reliable Are Large Language Models for Health Literacy? | Publish Date: November 30, 2023
Part 3 | When LLMs May Not Be Appropriate for Health Literacy | Publish Date: December 5, 2023
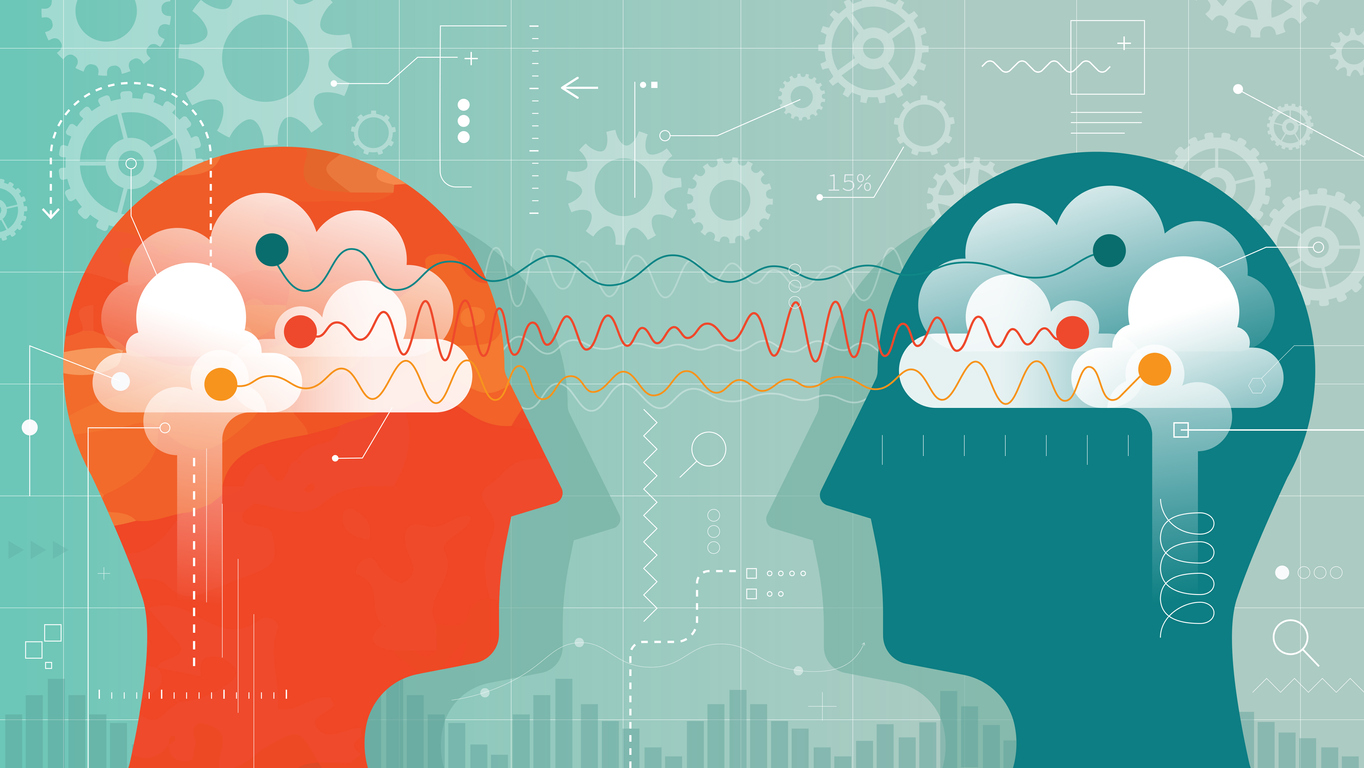
In our last post in this series, inspired by a recent discussion on the IHA Health Literacy Solutions Center discussion list about the implications of artificial intelligence (AI) for the health literacy field, I’ll discuss the road ahead for large language models (LLMs) for health literacy. I’ll begin with the current state of LLM evaluation and move to AI standards, regulation, and the role of the health literacy community.
The Current State of LLM Evaluation
To understand where things are now, I’ll start by establishing two key points:
-
LLM evaluation, and reporting on any evaluation, is currently entirely voluntary. Per our first post in this series, the voluntary nature of LLM evaluation and reporting on that evaluation doesn’t mean it isn’t happening. It does mean that there are no requirements for doing so or standards for exactly what gets evaluated or how. Again, as discussed in our first post, it’s up to the ethics, responsibility, and best practices of specific LLM developers.
-
LLMs are technologies. Therefore, metrics, benchmark data sets, evaluation criteria, standards, etc. for these technologies come from technologically focused sources, which include, but are not limited to:
-
Bodies and organizations that focus on and regulate technology, such as the National Institute of Standards and Technology (NIST); the National Academies of Sciences, Engineering, and Medicine (NASEM); the National Science Foundation (NSF); and, in the case of medical technology, the Food & Drug Administration (FDA).
-
Technical conferences, publications, communities, and competitions, such as those sponsored by the Association for Computational Linguistics (ACL), NeurIPS, the Association for Computational Machinery (ACM), the Institute of Electronics and Electronics Engineers (IEEE), and Kaggle, among others.
To consider the accuracy of LLMs for health literacy, we’re reliant on health literacy-appropriate benchmark data sets and supporting evaluation and assessment criteria, coupled with a solid understanding of health literacy-related needs and goals.
So far, I haven’t turned up a single benchmark data set or any evaluation criteria designed for health literacy needs specifically. (And, as noted in earlier posts, while benchmark data sets that encode clinical knowledge are available, they are limited and not necessarily a good fit for health literacy’s purposes.)
Things to Come: How Federal Regulation Will Play Out
Benchmark data sets and other forms of evaluation are not in and of themselves regulation. President Biden’s Executive Order at the end of October 2023 calling for AI regulation will change some, but certainly not all, of this moving forward.
The coming federal regulation of AI is focused on safety and risk, particularly to National Security and imminent personal safety and security, such as deep fakes. It is also centered on techniques like red teaming,1 but not benchmarking or other evaluation criteria across the AI development lifecycle.
The responsibility for enforcing this has been distributed across agencies, with a chunk being put on NIST to, among other things, develop “a companion resource to the AI Risk Management Framework, NIST AI 100-1, for generative AI.” This is a tall order, which will hamper NIST’s ability to make progress on other fronts.
The FDA is another key agency where AI for health literacy is concerned because it approves medical technology for purchase. The FDA is only in the early stages of talking about how to assess and evaluate LLM and other generative AI-based technologies. I suspect it will be a while before there’s meaningful evaluation there due to the complex and dynamic nature of these systems.
(Side note: the FDA is only responsible for technologies being approved for sale. If a health insurer or major healthcare system builds its own AI, it would not be subject to FDA approval even though it would impact many patients and their outcomes.)
So, given the current state of things and the long list of responsibilities outlined as part of the Executive Order, I expect everyone will be consumed with all this over the next three to nine months at least. AI evaluation of any kind will remain voluntary for the foreseeable future and come from sources outside of federal agencies.
The Role of the Health Literacy Community
Domain-specific LLM evaluation sets needed for health literacy purposes, especially any that might come from federal agencies, will likely take a back burner unless the health literacy community creates a sense of urgency around its needs.
If LLM standards or transparency around LLMs, whether voluntary or required, to support health literacy work are important, health literacy professionals must collaborate in — and contribute to — relevant initiatives and be vocal about health literacy efforts outside of health literacy channels. It’s important that we in the AI field understand your work and goals as well as the real barriers to LLM use in your field. This is how we’ll ensure the needs of health literacy are represented, and that suitable benchmarking data sets and other mechanisms, such as third-party audits and certifications, are developed.
How can the health literacy community participate? By doing the following with health literacy’s needs specifically in mind:
-
Call attention to the need for health literacy-specific training and evaluation sets for LLMs.
-
Endorse and accredit health literacy-specific LLM data sets, both for model training and evaluation.
-
Incentivize, fund the development of, and contribute to new health literacy-specific LLM benchmark data sets.
-
Develop relevant standards and regulations for using LLMs in health literacy.
-
Call for action, input, and recommendations about what we should be evaluating for health literacy’s needs.
Where Does All This Leave Us?
LLMs are already part of the healthcare environment. Doctors, educators, caregivers, patients, and others actively use them to help with health literacy-related needs, whether they are regulated or not, and whether or not they are officially adopted by the health literacy community. We all need to continue to understand what these tools do; how they behave; and where, whether, and how to integrate them into health literacy tools, tasks, and workflows.
Even with standards and regulations, will AI designers and developers continue to make unwitting choices without realizing they will result in poor, damaging, or untrustworthy results, even when we’ve made our best efforts? Sadly, yes.
Are we continuing to develop and adopt practices to help avoid these situations so that LLMs are more trustworthy? Absolutely. Are there concerns about the black-box nature of these tools? You bet. Are there concerns about the data we’re using? Yes.
Is the industry as a whole — and society at large — aware of all this and working to increase transparency, promote responsibility, and gain a better understanding of the inner workings of these tools so that they are more responsible and trustworthy? I firmly believe so.
While LLMs may not ever be deemed completely trustworthy for all things (and who is?), I believe that we will see a day when they are trustworthy enough for many more tasks than they are now. These tasks may very well include meeting health literacy needs in ways that are non-harmful and, in fact, beneficial, freeing up humans to provide the human touch.
1. As mentioned in our first post in this series, “red teaming” is when people, often subject matter experts, pose as adversaries of the system attempting to expose weaknesses, vulnerabilities, and problems.↩
About the Author
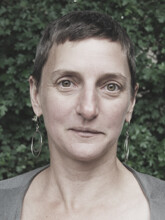
Temese Szalai has been an AI practitioner in the field of Natural Language Processing (NLP) and Machine Learning (ML) for over 20 years. She is the founder and principal of Subtextive, an independent woman-owned/woman-led provider of socio-technical AI consulting focused on AI initiatives for unstructured data for organizations large and small across a range of industries.
#ArtificialIntelligence
#IHABlog