Explained: AI + Health Literacy |
Can — and Should — We Trust Large Language Models for Health Literacy?
An Artificial Intelligence Practitioner’s Perspective on Trustworthiness and Responsibility, Part 1
By Temese Szalai | November 28, 2023
Read more in the series:
Part 2 | How Reliable Are LLMs for Health Literacy? | Publish Date: November 30, 2023
Part 3 | When LLMs May Not Be Appropriate for Health Literacy | Publish Date: December 5, 2023
Part 4 | Will LLMs Ever Really Be Trustworthy Enough for Health Literacy? | Publish Date: December 7, 2023
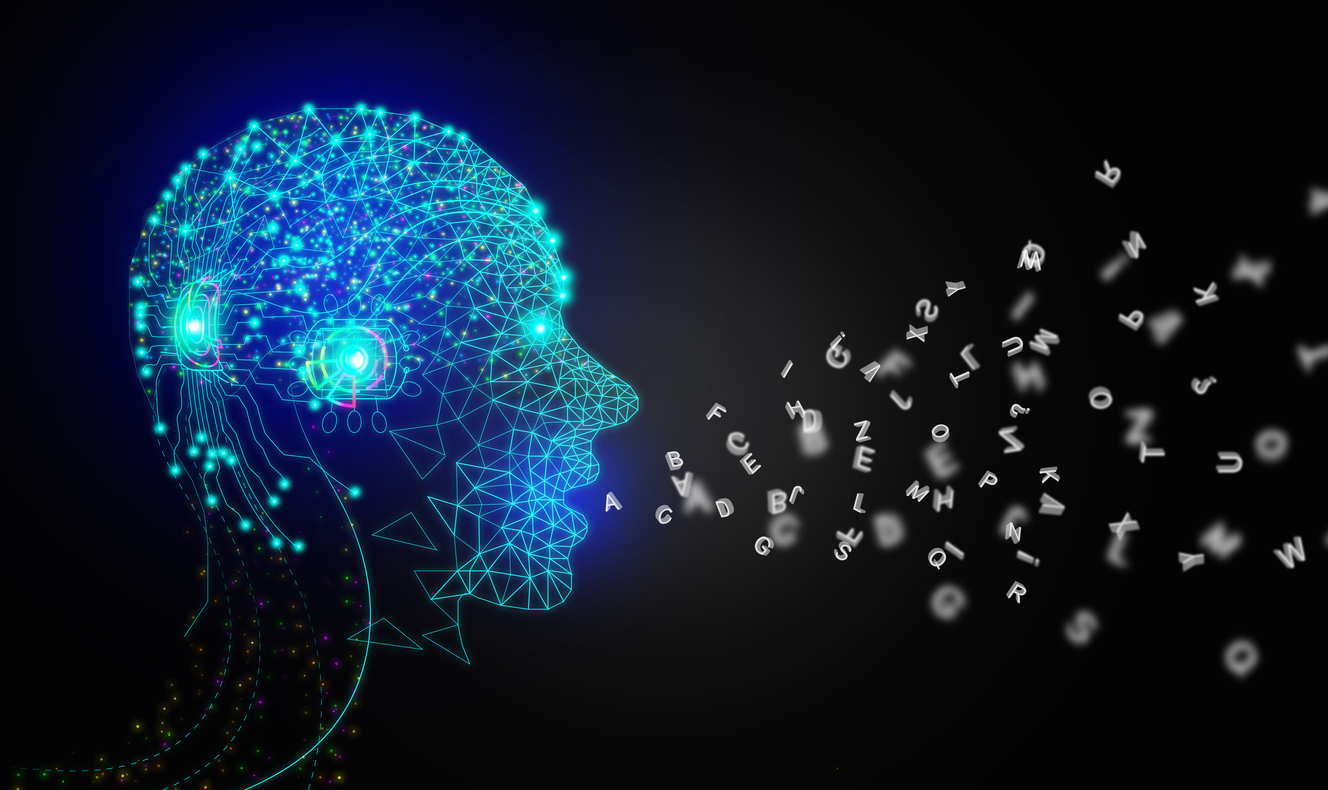
Since the release of OpenAI’s ChatGPT in November 2022, there’s been a seemingly incessant discussion about generative artificial intelligence (AI) powered by large language models (LLMs), sometimes referred to as chatbots. This four-part blog series focuses on considerations around the use of LLM-based AI, such as ChatGPT, for health literacy.
My comments here are largely in response to a thread on the Health Literacy Solutions Center discussion list prompted by the question: “AI is coming, like it or not! What does it mean for our work?” As a decades-long AI practitioner, now an AI strategy consultant, with expertise in natural language processing (NLP) and machine learning (ML), as well as an interest in health literacy, I wanted to offer my perspective on some of the major themes in this thoughtful discussion.
In this first post, I'll describe existing practices around responsibility in LLM development in the hopes of establishing more trust within health literacy work. In subsequent posts, I’ll:
-
Continue to explore trustworthiness of LLMs for health literacy by considering their ability to generate reliable health literate content.
-
Provide more insights on current — and possible future — practices to assess and ensure accuracy, usefulness, and fairness (i.e., responsibility) regarding LLM-generated content.
-
Look at the role and responsibility of those in the health literacy field in representing the interests of health literacy as AI technology and its evaluation evolve.
Working With a Moral Compass
The questions of trustworthiness and responsibility in AI are both huge and hugely important. At its core, and for purposes of this post, let’s define trustworthiness as whether someone — or something with some form of intelligence — can be counted on to be dependably honest and truthful. When we think about responsibility in AI, we’re thinking about accountability in AI’s development and use alongside supporting mechanisms to hold it accountable.
For starters, I want to stress that most of us in the field of AI work with a moral compass. We’re not unprincipled, unconscionable, or evil. We don’t intentionally build systems that aren’t reliable or trustworthy. And we do hold ourselves accountable.
With the advent of this new generation of AI technologies, I’ve seen an unprecedented amount of high-quality work and thought dedicated to mitigating the ethical, moral, and legal issues, as well as accuracy, relevancy, and other limitations of LLMs. The goals of these efforts are to strengthen and solidify practices for responsible development of these tools so that their outputs are more reliable and trustworthy for the purposes they are designed to achieve. A few examples include:
Credible and Medically Authoritative Evaluations
One question that arose on the Health Literacy Solutions Center discussion list is whether credible and medically authoritative evaluations of these programs exist. This question is both relevant and important because, generally, we trust systems when we know that they have passed tests to reliably complete certain tasks or generate specific kinds of outputs.
So, let’s break this question down into its constituent parts.
1. Are there credible evaluations of these programs?
Yes. AI is a professional field within science and engineering that holds itself to similar standards as other scientific disciplines.
That said, the AI field is far from perfect. Many in the field acknowledge there’s been some “bad science” to date, and there is a growing movement to do much better. This includes, among other things, scrutinizing existing methodologies, techniques, and metrics.
The field already utilizes a host of metrics that provide quantified, agreed-on, and objective means for measuring and assessing the performance of these technologies. Meanwhile, the industry is actively considering new metrics and ways to improve the application of existing metrics.
Evaluation methodologies, benchmarks, and measurement techniques continue to evolve to be more sophisticated, nuanced, and informative. Here are two methods worth mentioning:
2. How medically authoritative are these evaluations?
It depends. Most mass-market generalized LLMs, such as ChatGPT, Llama2, Claude, and Google Bard, don’t focus on medical authoritativeness per se. They don’t necessarily need to. The benchmarks they use are authoritative in relation to their design objective, which is general purpose.
That said, healthcare-specific LLMs do use medically authoritative evaluation sets. For example, Google’s Med-PaLM 2, a healthcare-specific LLM now being tested in clinics, was benchmarked against several independent third-party test sets, including PubMedQA, MedQA (USMLE), and MMLU (Massive Multitask Language Understanding) Clinical Topics. These results have been published to boot.
Benchmark data sets like these are medically authoritative, though not necessarily applicable to health literacy efforts or all healthcare needs across the board. Benchmark data sets that capture clinical knowledge are also relatively limited. So, there’s a need for more of them and with greater variety.
Does this mean LLMs are trustworthy for health literacy? Not necessarily.
Does it mean they aren’t trustworthy for health literacy? Also, not necessarily.
What it does mean is that we have some foundations, albeit perhaps a little shaky, on which to build trust, and a starting place for a conversation about what the health literacy field needs to trust LLMS. In our next post, I’ll continue this theme and talk about the reliability of LLM outputs for health literacy, which also impacts trustworthiness for health literacy efforts.
1. “Red teaming” is when people, often subject matter experts, pose as adversaries of the system attempting to expose weaknesses, vulnerabilities, and problems.↩
About the Author
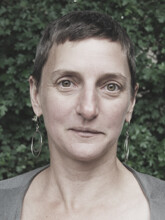
Temese Szalai has been an AI practitioner in the field of Natural Language Processing (NLP) and Machine Learning (ML) for over 20 years. She is the founder and principal of Subtextive, an independent woman-owned/woman-led provider of socio-technical AI consulting focused on AI initiatives for unstructured data for organizations large and small across a range of industries.
#IHABlog
#ArtificialIntelligence